What is Predictive Maintenance?
Predictive maintenance (PdM), is about enabling needs-based and condition-based maintenance, rather than maintenance that may be required. The keyword here is “maintenance requirements”. Traditional maintenance measures involve fixed maintenance schedules and reactive measures in the event of system failures. This often results in unnecessary downtime and high costs. Predictive maintenance and facility management are terms that are often used in conjunction.
PdM uses historical and real-time data to predict the optimal time for maintenance work and minimize unplanned machine downtime with the help of AI, machine learning, data analysis, and monitoring technology. The maintenance measures are adapted to the requirements of the individually monitored systems.
Preventive predictive maintenance (PPM) is a combination of two approaches to maintenance: preventive maintenance and predictive maintenance. PPM uses real-time data and predictive analytics to inform and optimize the schedule of preventive maintenance tasks. This means that maintenance activities are not just based on fixed schedules but are dynamically adjusted based on the actual condition and performance of the equipment.
How Does Predictive Maintenance Work?
IoT sensor technologies capture relevant status data such as vibrations, temperature, or power consumption. MQTT (Message Queuing Telemetry Transport) is a lightweight, publish-subscribe-based messaging protocol that is often used in the context of the Internet of Things (IoT). IoT sensors act as MQTT clients and regularly publish their measurement data to specific topics on the MQTT broker.
All data collected by the IoT sensors is transferred to cloud or edge computing platforms. The data is stored and analyzed there. Cloud computing platforms enable scalability and accessibility. Edge computing platforms enable data to be processed closer to the source and in real time. Predictive algorithms and data analysis are then used to identify patterns that indicate impending failures. 5G predictive maintenance involves using a 5G network to enable reliable high-volume data transmission.
Machine learning (ML) and artificial intelligence (AI) algorithms and statistical models are then used to plan an optimum maintenance interval. The infrastructure of predictive maintenance solutions includes data acquisition and storage, data processing, condition monitoring for maintenance, forecasting and asset health evaluation.
Digital twins of physical equipment can also be created based on the data collected by IoT sensors. This allows companies to simulate real-life scenarios in order to optimize processes by making better decisions. By integrating predictive maintenance into the Industry 4.0 framework, companies can create a highly efficient, flexible and reliable production environment.
Wireless IoT Technologies and Predictive Maintenance
Products for Predictive Maintenance
There are two main components for a predictive maintenance system based on IoT technology. The first is the hardware. This includes IoT devices such as sensors, smart meters, IoT gateways, actuators, and programmable logic controllers (PLCs) that collect data. These devices are attached to machines and throughout the production line. Sensors are the core components for predictive maintenance. There are different types of sensors that measure different parameters such as vibration, temperature, pressure, and noise. Data acquisition systems are used to ensure that all collected data is transmitted and processed for analysis. From here, connectivity devices forward the data processed by the data acquisition systems to a central cloud storage or analysis software. Transmission can take place via wired networks, mobile networks such as 5G or Wi-Fi. OPC UA can be used to ensure interoperable communication between different sensors and devices from different manufacturers.
The second component is the data analysis software for processing and analyzing the collected data. This includes AI and machine learning algorithms, for example. AI in companies enables the prediction of plant downtimes based on the analyzed data. In other words, it transforms raw data into actionable insights. Enterprise resource planning (ERP) systems and computerized maintenance management systems (CMMS) can also be integrated with data analytics software.
In addition to the maintenance management software, software components such as predictive modeling are also used. This software acts as an operational hub for the management of maintenance activities.
Facts & Figures
Maintenance work is expensive. According to a research paper by Newcastle University, 15 to 40 percent of production costs are spent on maintenance. Predictive maintenance can help to reduce these costs. According to a report by “Deloitte Insights”, a company that conducts its own research and in-depth analysis, companies that use predictive maintenance solutions can reduce overall maintenance costs by 5-10 percent.
The time required for planning maintenance work can also be reduced by 20-50 percent. Plant availability and up-time can be increased by 10-20 percent. In addition to these benefits, predictive maintenance can reduce machine and equipment downtime by 35 to 50 percent and extend the life of machines and equipment by 20 to 40 percent, according to a report by “Nucleus Research”, a provider of ROI-oriented technology research.
Successful Examples of Predictive Maintenance
Predictive maintenance strategies are used in many industries. These include: Manufacturing and production, the energy industry, transportation, logistics, oil and gas, mining, aerospace, construction, and chemical and pharmaceutical industries. Companies that use these strategies save costs, reduce machine and plant downtimes, and increase the safety of machine use. This optimizes operational processes along the supply chain. The following practical examples from industry and the energy sector show how wireless IoT technologies are used for predictive maintenance.
Predictive Maintenance at Rosendahl Nextrom
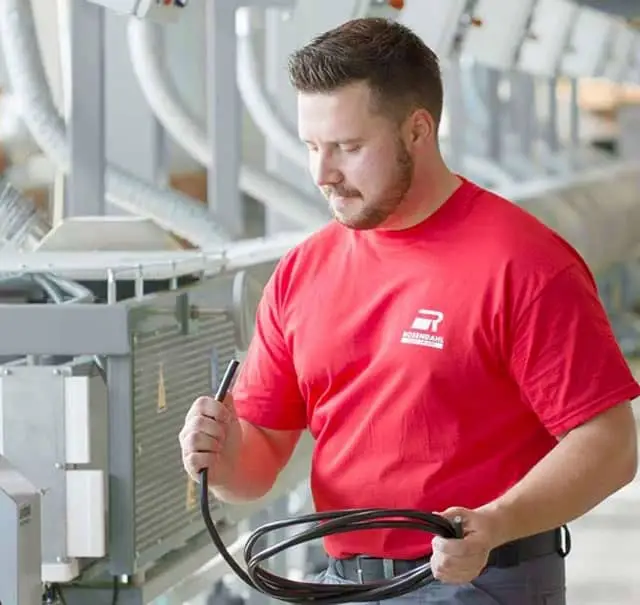
Rosendahl Nextrom, a manufacturer of cables, fiber optics, and batteries, uses the OPC UA communication standard from the OPC Foundation for the predictive maintenance of its plants. In the extruder section of the plant, for example, tensile stress, temperature, and object data captured during condition monitoring is transmitted via OPC UA. Predictive maintenance is made possible by analyzing empirical values and historical data. The result: an optimized service life of the installed screws.
Rosendahl Nextrom, a manufacturer of cables, fiber optics, and batteries, uses the OPC UA communication standard from the OPC Foundation for the predictive maintenance of its plants. In the extruder section of the plant, for example, tensile stress, temperature, and object data captured during condition monitoring is transmitted via OPC UA. Predictive maintenance is made possible by analyzing empirical values and historical data. The result: an optimized service life of the installed screws.
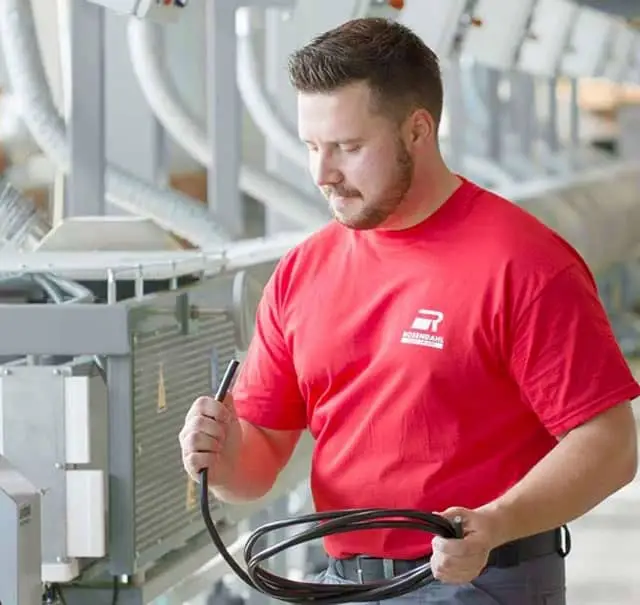
“The biggest advantage is, of course, the easier connection of machines to existing systems. By eliminating hardware adapters, we become more independent and flexible. Conversely, the standard also enables the availability of new process values and comprehensive data access in real time.”
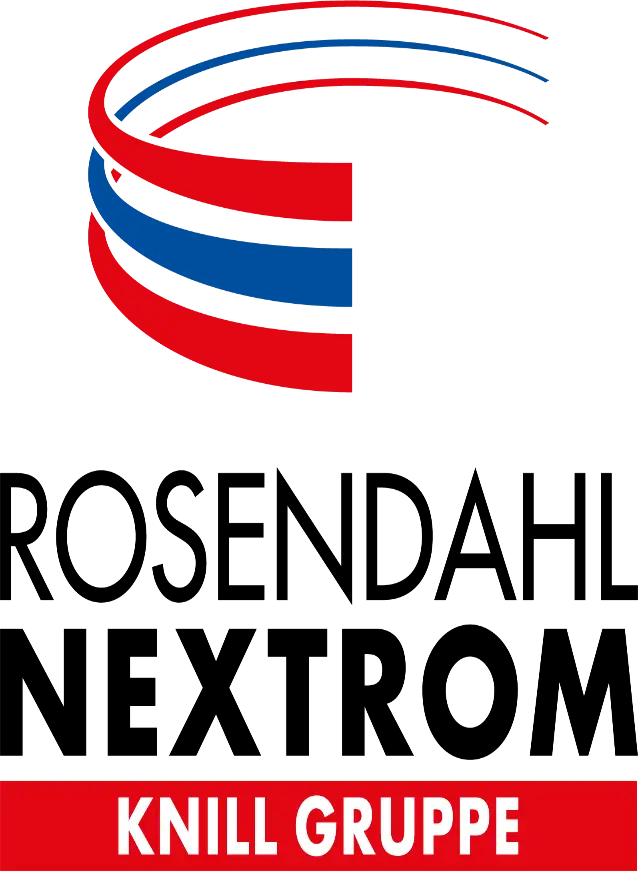
Benedikt Wagner
Head of Commissioning and Programming
Predictive Maintenance at Scatec
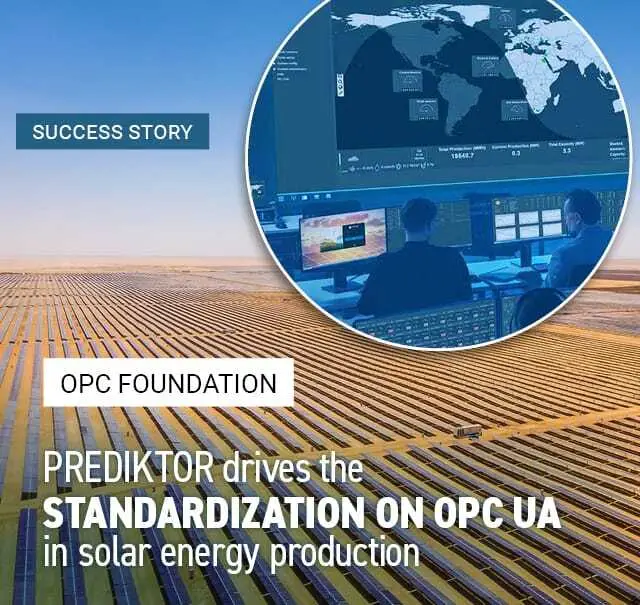
The solar power provider Scatec uses “PowerView” for data management. PowerView is a data-driven solution based on the OPC UA standard of the OPC Foundation. Over 500,000 solar panels are maintained by keeping them clean to avoid losses and performance problems due to soiling. PowerView generates suggestions for optimal cleaning and maintenance times based on device and system data, as well as contextual data such as weather forecasts. The result: Reduced maintenance costs and improved resource planning.
The solar power provider Scatec uses “PowerView” for data management. PowerView is a data-driven solution based on the OPC UA standard of the OPC Foundation. Over 500,000 solar panels are maintained by keeping them clean to avoid losses and performance problems due to soiling. PowerView generates suggestions for optimal cleaning and maintenance times based on device and system data, as well as contextual data such as weather forecasts. The result: Reduced maintenance costs and improved resource planning.
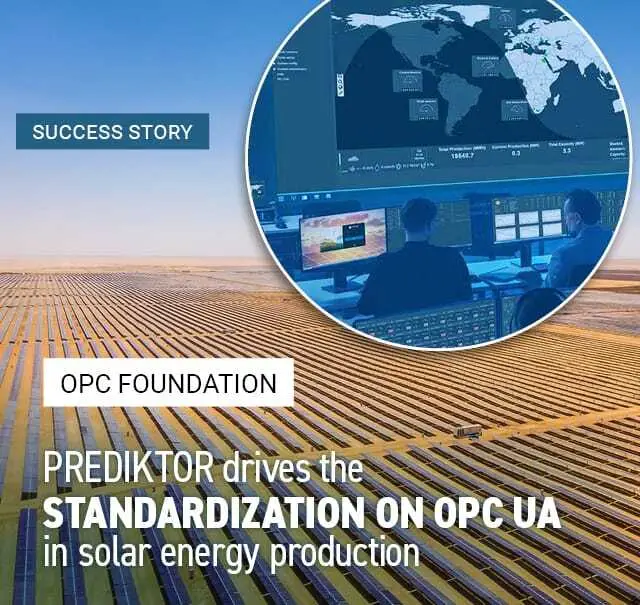
“Without such a system, the problem arises that the collection of more and more data does not necessarily generate added value. The operator is overloaded with data. For example, hundreds of alarms can be displayed without having an appropriate response ready. What is needed is a system that can dive into the data streams, find out what is causing the alarms, and make high-quality recommendations on how to resolve the problems.”
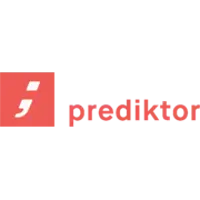
Thomas Pettersen
Vice President Operations Management
Predictive Maintenance at Groupe Renault
Groupe Renault uses the OPC UA communication standard from the OPC Foundation for the automated welding of components. The use of adapters makes components such as welding processes, robots, CNC, and PLCs OPC UA-capable. The 2,200 connected robots in the body shop monitor welding processes, detect anomalies in real time, and carry out predictive maintenance on the components based on the data collected. The result: A 20 percent reduction in the error rate in the welding process.
More Stories on Predictive Maintenance
The Future of Predictive Maintenance
The future of predictive maintenance will be characterized by a combination of technological advances and strategic approaches. The aim is to further improve the efficiency, reliability, and sustainability of maintenance processes. Predictive maintenance will be an integral part of Industry 4.0 and Manufacturing X initiatives. It will be seamlessly integrated with smart factories, where interconnected systems and advanced automation will ensure optimal performance and minimal downtime.
With the development of algorithms for artificial intelligence and machine learning, more accurate predictions of machine failures will be possible. Predictive analytics uses these algorithms to analyze large amounts of data in order to identify patterns and trends in the condition of machines. Cloud computing will enable centralized data repositories where vast amounts of maintenance data can be stored and accessed globally. This will facilitate collaboration and data sharing across different locations and departments, improving overall maintenance strategies. As the volume of data collected increases, big data analytics will be required to handle and analyze massive datasets collected from IoT devices.
Predictive maintenance is also becoming more and more virtual. Proactive maintenance strategies are increasingly made possible through the use of digital twins. The condition of physical systems can be monitored virtually and in real time. AR-based solutions for predictive maintenance are being used more and more frequently. The aim here is to simulate real-life scenarios in which maintenance staff can safely practice their skills. Virtual reality technologies in predictive maintenance are used to virtually represent either the plant itself or specific parts, components, or products. These can either be existing objects or objects that do not yet exist but are planned. Extended reality (XR) is an emerging trend in plant inspection. This involves the integration of augmented and virtual reality tools into maintenance processes. Remote support, immersive training, and virtual simulations in the field of predictive maintenance are made possible by XR technologies like AR and VR.
Another buzzword for the future of predictive maintenance is “real-time”. A combination of edge computing and predictive analytics is used to enable predictive maintenance in real time. This combines artificial intelligence and business intelligence (BI) to analyze the condition of the system. More and more companies are recognizing the potential of edge computing solutions for predictive maintenance: sensor data can be analyzed in real time to facilitate and accelerate decision-making for maintenance work.
The future of predictive maintenance will also involve the collaboration between humans and machines (human-robot collaboration / HRC). Advanced interfaces and AI-driven insights will assist technicians in making informed decisions, while machines handle routine diagnostics and maintenance tasks. Collaborative robots, or cobots, will work alongside human technicians, assisting with complex maintenance tasks and ensuring precision. These robots will be equipped with AI and IoT capabilities to perform real-time diagnostics and repairs.
Advantages of IoT-Based Predictive Maintenance
Advantages of Wireless IoT
- Real-time monitoring
- Cost reduction
- Avoidance of production downtimes
- Extended service life of the machines
- Data-driven decisions
Scientific research has shown that it is crucial for companies to prioritize the reliability of machines. Predictive maintenance solutions play a crucial role here, especially in conjunction with IoT technologies. There are five main benefits of implementing IoT predictive maintenance:
- By detecting potential problems at an early stage, production downtime and the associated losses can be avoided. As maintenance work is only carried out when it is actually needed, machine up-time is maximized.
- By avoiding unplanned downtime and minimizing maintenance work that is not required, maintenance costs are significantly reduced.
- With IoT-based predictive maintenance, maintenance processes are better planned and coordinated, resulting in more efficient use of resources and personnel.
- The continuous monitoring of a machine's condition identifies and rectifies potential safety risks at an early stage, thereby increasing safety in the workplace. This means that the risk of breakdowns leading to accidents is lower, which in turn increases occupational safety for maintenance staff.
- Machines that are optimally maintained work more precisely and reliably, which improves the quality of the goods produced. With the right maintenance plans, the service life of machines and systems is extended.
Scientific research has shown that it is crucial for companies to prioritize the reliability of machines. Predictive maintenance solutions play a crucial role here, especially in conjunction with IoT technologies. There are five main benefits of implementing IoT predictive maintenance:
- By detecting potential problems at an early stage, production downtime and the associated losses can be avoided. As maintenance work is only carried out when it is actually needed, machine up-time is maximized.
- By avoiding unplanned downtime and minimizing maintenance work that is not required, maintenance costs are significantly reduced.
- With IoT-based predictive maintenance, maintenance processes are better planned and coordinated, resulting in more efficient use of resources and personnel.
- The continuous monitoring of a machine's condition identifies and rectifies potential safety risks at an early stage, thereby increasing safety in the workplace. This means that the risk of breakdowns leading to accidents is lower, which in turn increases occupational safety for maintenance staff.
- Machines that are optimally maintained work more precisely and reliably, which improves the quality of the goods produced. With the right maintenance plans, the service life of machines and systems is extended.
Advantages of Wireless IoT
- Real-time monitoring
- Cost reduction
- Avoidance of production downtimes
- Extended service life of the machines
- Data-driven decisions
Challenges of IoT-Based Predictive Maintenance
Implementing IoT-based predictive maintenance systems can offer significant benefits, but it also requires overcoming numerous challenges. Careful planning, appropriate security measures, continuous monitoring and adjustments, as well as the training of skilled personnel are crucial for the success of such projects.
There are three main challenges when implementing an IoT-based predictive maintenance system.
1. Technical Challenges
Different machines and devices often use different protocols and standards, which makes system integration and interoperability difficult. To solve this problem, companies need to use interoperable communication standards such as OPC UA.
IoT sensors generate enormous amounts of data that need to be processed and analyzed. High data quality and accurate data collection are crucial in order to make reliable predictions. Managing and processing such large amounts of data is also a challenge. This requires sufficient bandwidth, storage, and computing resources. Companies must therefore invest in an infrastructure for data management.
Systems must also be scalable in order to keep pace with the growing volume of data and the number of networked devices.
2. Organizational Challenges
The implementation of IoT-based PdM systems can require significant initial investment in hardware, software, and infrastructure. There is also a need for skilled professionals who have both technical knowledge of IoT and data analytics as well as maintenance expertise. PdM systems need to be seamlessly integrated into existing IT and maintenance systems, which can be complex and time-consuming. The introduction of new technologies in maintenance can also be a challenge for employees. This is mainly due to a lack of trust and understanding of new technologies. For this reason, appropriate training and support are crucial for the successful introduction of IoT-based predictive maintenance solutions.
3. Challenges in Security and Data Protection
IoT devices and networks are vulnerable to cyberattacks. It is important to implement robust security measures to protect data and systems. Cybersecurity solutions include regular security audits, intrusion detection systems, and network segmentation. The collection and processing of large amounts of operational data also raises data protection issues. It must be ensured that all legal requirements and data protection guidelines are complied with.
Partners Spezialized in Predictive Maintenance Solutions
Outlook – Next-Level Predictive Maintenance
Some of the emerging trends in predictive maintenance include faster and more cost-effective implementation through Predictive Maintenance-as-a-Service (PdMaaS), improved workplace safety through robot-based inspections, and the use of immersive technologies such as extended reality.
Predictive Maintenance-as-a-Service
Predictive Maintenance-as-a-Service (PdMaaS) involves the combination of software and a service team to make predictive maintenance more easily available, accessible, and affordable for manufacturing companies and facilities. There is minimal disruption to the implementation process, as little input is required from factory or plant staff. No training is required. PdM-as-a-Service can also be customized to the specific requirements of plants and factories.
Inspections with Autonomous Robots
In hazardous areas, such as the oil and gas industry, companies are increasingly using automated robot inspections to monitor facilities. Autonomous robots and drones are used as mobile sensor platforms. These are used to monitor plants. The data is then transferred to computerized maintenance management systems (CMMS).
Immersive Technologies
Predictive maintenance solutions using extended reality technologies like augmented and virtual reality, are being further developed to enable remote maintenance monitoring, improve maintenance visualization and enhance remote support and training for maintenance staff.